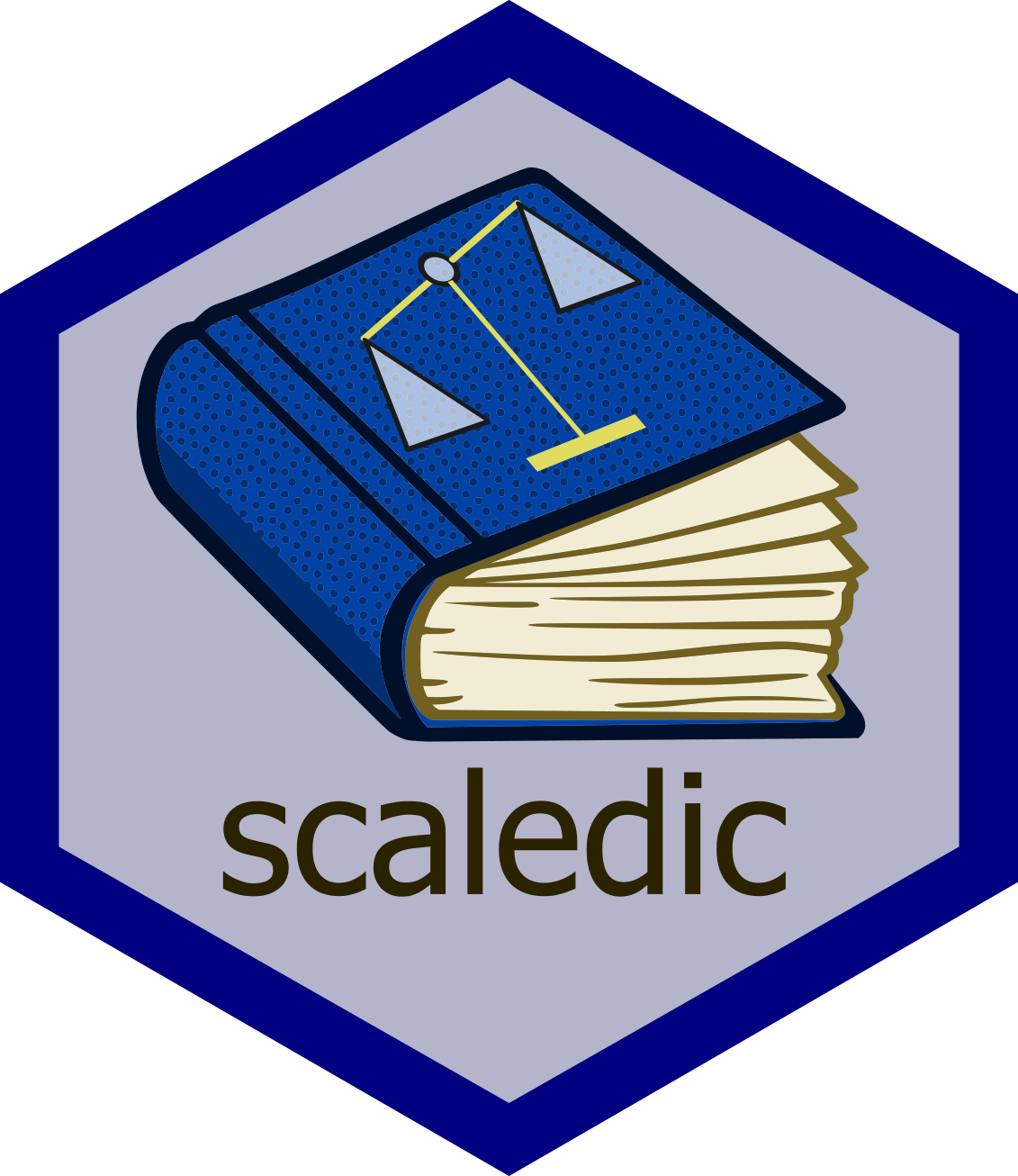
Introduction to scaledic
scaledic.rmd
What is a dictionary file?
When you conduct research based on questionnaires or psychometric tests (and you are working in R), you typically create a data.frame with one column (variable) for each item on that questionnaire and one row for each person who participated. You can only store a limited amount of additional information about each item in that questionnaire within a data.frame (or tibble). You can give a variable a name and define a variable as a factor with appropriate levels. But basically, that is it. You cannot, at least not conveniently, include a longer label for each item, the name of a scale to which that item belongs to, information about reverse coding, etc.
I call the collection of this additional information about items an item dictionary. A dictionary contains a short label, a longer description, scale affiliation, and more for each item.
A dictionary file
A dictionary file is a table with one row for each variable and one column for each attribute of those variables. The most convenient way to create a dictionary file is in a spreadsheet program for later use with data sets.
Here is an extract from an example dic-file:
item_name | item_label | scale | scale_label | subscale | subscale_label | values | value_labels | missing | type |
---|---|---|---|---|---|---|---|---|---|
itrf_I_1 | Verbringt zu viel Zeit alleine | ITRF | Integrated teacher report form | Int | Internalizing | 0:3 | 0 = not problematic; 1 = slightly problematic; 2 = problematic; 3 = strongly problematic | -99 | integer |
itrf_I_2 | Beschwert sich über Krankheit oder Schmerzen | ITRF | Integrated teacher report form | Int | Internalizing | 0:3 | 0 = not problematic; 1 = slightly problematic; 2 = problematic; 3 = strongly problematic | -99 | integer |
itrf_I_4 | Vermeidet soziale Interaktionen | ITRF | Integrated teacher report form | Int | Internalizing | 0:3 | 0 = not problematic; 1 = slightly problematic; 2 = problematic; 3 = strongly problematic | -99 | integer |
A dictionary file can contain any additional attributes. This means that you can add a column with any name to store relevant information (e.g. the scale and scale label to which an item belongs, a translation of the item name). However, there are some predefined attributes with a specific meaning. The table below shows these attributes:
Parameter | Meaning | Example |
---|---|---|
item_name | A short item name | itrf_1 |
item_label | Full text of the item | Vermeidet die Teilnahme an Diskussionen im Unterricht |
values | Valid response values in an R manner | 1:5 (for integers 1 to 5) 1,2,3 (for integers 1, 2, 3) |
value_labels | Labels for each response value | 0 = nicht; 1 = leicht; 2 = mäßig; 3 = stark |
missing | Missing values | -888, -999 |
type | Data type (factor, integer, float, real) | integer |
weight | Reversion of item and its weight | 1 (positive), -1 (reverse), 1.5 (positive, weights 1.5 times) |
Apply a dictionary file
When you combine a dataset with a dictionary file, each variable in
the dataset that corresponds to a variable described in the dictionary
is completed with the given dictionary information.
The resulting dataset is now ready for use with all other
scaledic
functions.
The apply_dic
function takes the name of the dataset and
the dictionary file and combines them. Missing values are replaced by
NAs:
# Here we use the example dataset "dat_itrf" and the example dic file "dic_itrf"
dat <- apply_dic(dat_itrf, dic_itrf)
Let us take a look at all the scales in the dataset:
list_scales(dat, paste0(c("scale", "subscale", "subscale_2"), "_label")) %>% kable()
scale_label | subscale_label | subscale_2_label | |
---|---|---|---|
itrf_I_1 | Integrated teacher report form | Internalizing | Socially Withdrawn |
itrf_I_2 | Integrated teacher report form | Internalizing | Anxious/Depressed |
itrf_I_20 | Integrated teacher report form | Externalizing | Oppositional/Disruptive |
itrf_E_1 | Integrated teacher report form | Externalizing | Academic Productivity/Disorganization |
Clean raw data
Firstly, we check for invalid values in the dataset (e.g., typos) and replace them with NA:
dat <- check_values(dat, replace = NA)
Now we impute missing values:
# Imputation for items of the subscale Ext
dat <- impute_missing(dat, subscale == "Ext")
# Imputation for items of the subscale Int
dat <- impute_missing(dat, subscale == "Int")
Select scales for analyszing
Let us look at the descriptive statistics for the internalising subscale:
dat %>%
select_items(subscale == "Int") %>%
descriptives(round = 1)
#> name valid missing mean sd min max range median mad
#> 1 itrf_I_1 4772 4 0.4 0.7 0 3 3 0 0
#> 2 itrf_I_2 4772 4 0.3 0.7 0 3 3 0 0
#> 3 itrf_I_4 4772 4 0.3 0.6 0 3 3 0 0
#> 4 itrf_I_5 4772 4 0.2 0.6 0 3 3 0 0
#> 5 itrf_I_6 4772 4 0.2 0.5 0 3 3 0 0
#> 6 itrf_I_7 4772 4 0.4 0.7 0 3 3 0 0
#> 7 itrf_I_8 4772 4 0.3 0.7 0 3 3 0 0
#> 8 itrf_I_9 4772 4 0.5 0.8 0 3 3 0 0
#> 9 itrf_I_10 4772 4 0.3 0.7 0 3 3 0 0
#> 10 itrf_I_11 4772 4 0.3 0.7 0 3 3 0 0
#> 11 itrf_I_12 4772 4 0.4 0.7 0 3 3 0 0
#> 12 itrf_I_13 4772 4 0.4 0.7 0 3 3 0 0
#> 13 itrf_I_14 4772 4 0.3 0.7 0 3 3 0 0
#> 14 itrf_I_15 4772 4 0.4 0.7 0 3 3 0 0
#> 15 itrf_I_16 4772 4 0.4 0.8 0 3 3 0 0
#> 16 itrf_I_17 4772 4 0.4 0.7 0 3 3 0 0
#> 17 itrf_I_19 4772 4 0.2 0.6 0 3 3 0 0
#> 18 itrf_I_23 4772 4 0.4 0.7 0 3 3 0 0
#> 19 itrf_I_24 4772 4 0.4 0.7 0 3 3 0 0
See items instead of labels
It is more convenient to see the original items rather than the short labels:
dat %>%
select_items(subscale == "Int") %>%
rename_items() %>%
descriptives(round = 1) %>%
kable()
name | valid | missing | mean | sd | min | max | range | median | mad |
---|---|---|---|---|---|---|---|---|---|
Verbringt zu viel Zeit alleine | 4772 | 4 | 0.4 | 0.7 | 0 | 3 | 3 | 0 | 0 |
Beschwert sich über Krankheit oder Schmerzen | 4772 | 4 | 0.3 | 0.7 | 0 | 3 | 3 | 0 | 0 |
Vermeidet soziale Interaktionen | 4772 | 4 | 0.3 | 0.6 | 0 | 3 | 3 | 0 | 0 |
Spielt bevorzugt alleine | 4772 | 4 | 0.2 | 0.6 | 0 | 3 | 3 | 0 | 0 |
Geht nicht auf Kontaktversuche der Mitschülerinnen und Mitschüler ein | 4772 | 4 | 0.2 | 0.5 | 0 | 3 | 3 | 0 | 0 |
Macht sich Sorgen über unwichtige Details | 4772 | 4 | 0.4 | 0.7 | 0 | 3 | 3 | 0 | 0 |
Beschwert sich über Kopfschmerzen oder Bauchschmerzen | 4772 | 4 | 0.3 | 0.7 | 0 | 3 | 3 | 0 | 0 |
Wirkt unglücklich oder traurig | 4772 | 4 | 0.5 | 0.8 | 0 | 3 | 3 | 0 | 0 |
Klammert sich an Erwachsene | 4772 | 4 | 0.3 | 0.7 | 0 | 3 | 3 | 0 | 0 |
Verhält sich nervös | 4772 | 4 | 0.3 | 0.7 | 0 | 3 | 3 | 0 | 0 |
Verhält sich ängstlich | 4772 | 4 | 0.4 | 0.7 | 0 | 3 | 3 | 0 | 0 |
Behauptet sich nicht gegenüber anderen | 4772 | 4 | 0.4 | 0.7 | 0 | 3 | 3 | 0 | 0 |
Verhält sich übermäßig schüchtern | 4772 | 4 | 0.3 | 0.7 | 0 | 3 | 3 | 0 | 0 |
Beklagt sich oder jammert | 4772 | 4 | 0.4 | 0.7 | 0 | 3 | 3 | 0 | 0 |
Beteiligt sich nicht an Gruppenaktionen | 4772 | 4 | 0.4 | 0.8 | 0 | 3 | 3 | 0 | 0 |
Macht sich selbst schlecht | 4772 | 4 | 0.4 | 0.7 | 0 | 3 | 3 | 0 | 0 |
Weint oder ist weinerlich | 4772 | 4 | 0.2 | 0.6 | 0 | 3 | 3 | 0 | 0 |
Macht sich ständig Sorgen | 4772 | 4 | 0.4 | 0.7 | 0 | 3 | 3 | 0 | 0 |
Lässt sich langsam auf neue Personen ein | 4772 | 4 | 0.4 | 0.7 | 0 | 3 | 3 | 0 | 0 |
And then we analyse the factor structure. Here we use the
rename_item()
function to get a more convenient
description.
dat %>%
select_items(scale == "ITRF") %>%
rename_items(pattern = "({reverse}){subscale}_{subscale_2}: {label}", max_chars = 70) %>%
exploratory_fa(nfactors = 4, cut = 0.4) %>% kable()
MR1 | MR3 | MR2 | MR4 | |
---|---|---|---|---|
(+)Ext_OPP: Verliert die Beherrschung | 0.85 | |||
(+)Ext_OPP: Macht unangebrachte Bemerkungen | 0.83 | |||
(+)Ext_OPP: Streitet und zankt mit Lehrkräften | 0.8 | |||
(+)Ext_OPP: Hat Konflikte mit Mitschülerinnen und Mitschülern | 0.8 | |||
(+)Ext_OPP: Kommandiert rum | 0.78 | |||
(+)Ext_OPP: Verwendet unangemessene Sprache | 0.78 | |||
(+)Ext_OPP: Ist schnell verärgert | 0.76 | |||
(+)Ext_OPP: Stört andere | 0.65 | |||
(+)Ext_OPP: Respektiert nicht die Privatsphäre anderer | 0.64 | |||
(+)Ext_APD: Erledigt Hausaufgaben unvollständig | 0.82 | |||
(+)Ext_APD: Zeigt Unterrichtsaufgaben nicht selbstständig vor | 0.81 | |||
(+)Ext_APD: Stellt Unterrichtsaufgaben nicht rechtzeitig fertig | 0.76 | |||
(+)Ext_APD: Kommt unvorbereitet zum Unterricht | 0.73 | |||
(+)Ext_APD: Kontrolliert seine eigene Arbeit nicht | 0.73 | |||
(+)Ext_APD: Nimmt Materialien, die zu Hause benötigt werden, nicht mit | 0.72 | |||
(+)Ext_APD: Beginnt mit der Aufgabenbearbeitung nicht selbstständig | 0.72 | |||
(+)Ext_APD: Beteiligt sich nicht am Unterricht | 0.53 | |||
(+)Int_SW: Vermeidet soziale Interaktionen | 0.86 | |||
(+)Int_SW: Geht nicht auf Kontaktversuche der Mitschülerinnen und Mits | 0.79 | |||
(+)Int_SW: Spielt bevorzugt alleine | 0.78 | |||
(+)Int_SW: Verbringt zu viel Zeit alleine | 0.66 | |||
(+)Int_SW: Beteiligt sich nicht an Gruppenaktionen | 0.66 | |||
(+)Int_SW: Verhält sich übermäßig schüchtern | 0.55 | |||
(+)Int_SW: Behauptet sich nicht gegenüber anderen | 0.5 | |||
(+)Int_SW: Lässt sich langsam auf neue Personen ein | 0.47 | |||
(+)Int_AD: Beschwert sich über Kopfschmerzen oder Bauchschmerzen | 0.73 | |||
(+)Int_AD: Macht sich ständig Sorgen | 0.71 | |||
(+)Int_AD: Beschwert sich über Krankheit oder Schmerzen | 0.7 | |||
(+)Int_AD: Beklagt sich oder jammert | 0.65 | |||
(+)Int_AD: Macht sich Sorgen über unwichtige Details | 0.65 | |||
(+)Int_AD: Weint oder ist weinerlich | 0.59 | |||
(+)Int_AD: Verhält sich ängstlich | 0.47 | |||
(+)Int_AD: Wirkt unglücklich oder traurig | 0.47 | |||
(+)Int_AD: Macht sich selbst schlecht | 0.44 | |||
(+)Int_AD: Klammert sich an Erwachsene | 0.4 | |||
(+)Int_AD: Verhält sich nervös | 0.4 | |||
SS loadings | 6 | 4.79 | 4.53 | 4.35 |
Proportion Var | 0.17 | 0.13 | 0.13 | 0.12 |
Cumulative Var | 0.17 | 0.3 | 0.43 | 0.55 |
Proportion Explained | 0.31 | 0.24 | 0.23 | 0.22 |
Cumulative Proportion | 0.31 | 0.55 | 0.78 | 1 |
and provide item analyses
scales <- ex_itrf %>% get_scales(
'APD' = subscale_2 == "APD",
'OPP' = subscale_2 == "OPP",
"SW" = subscale_2 == "SW",
"AD" = subscale_2 == "AD"
)
alpha_table(dat, scales = scales) %>% kable()
Scale | n | n items | Alpha CI95% | Std.Alph CI95% | Homogeneity | Discriminations | Means | SDs | |Loadings| |
---|---|---|---|---|---|---|---|---|---|
APD | 4776 | 8 | .91 [.90, .91] | .91 [.91, .91] | .56 | [.53, .78] | [0.38, 0.95] | [0.75, 1.04] | [.55, .82] |
OPP | 4776 | 9 | .94 [.93, .94] | .94 [.94, .94] | .63 | [.68, .81] | [0.35, 0.83] | [0.72, 0.96] | [.70, .85] |
SW | 4772 | 8 | .88 [.87, .88] | .88 [.88, .89] | .48 | [.53, .78] | [0.21, 0.43] | [0.51, 0.76] | [.56, .86] |
AD | 4772 | 11 | .88 [.88, .89] | .88 [.88, .89] | .41 | [.52, .69] | [0.23, 0.48] | [0.59, 0.77] | [.55, .74] |
Build scale scores
Now we will create scores for the internalizing and externalizing scales.
dat$itrf_ext <- score_scale(dat, scale == "ITRF" & subscale == "Ext", label = "Externalizing")
dat$itrf_int <- score_scale(dat, scale == "ITRF" & subscale == "Int", label = "Internalizing")
and get descriptives for those scores
dat %>%
select_scores() %>%
rename_items() %>%
descriptives(round = 1)
#> name valid missing mean sd min max range median mad
#> 1 Internalizing 4772 4 0.3 0.4 0 2.6 2.6 0.2 0.3
#> 2 Externalizing 4776 0 0.6 0.6 0 3.0 3.0 0.4 0.5
Look up norms from a norm table
Many scales come with norm tables to convert raw scores to t-scores, percentile ranks, etc.
The lookup_norms
function helps with this
conversion.
Firstly, you need a data frame (or Excel table etc) which includes raw-scores and corresponding norm-scores.
Here is an example of such a table:
group | raw | T | PR | T_from_PR |
---|---|---|---|---|
all | 0 | 42 | 26 | 43 |
all | 1 | 43 | 35 | 46 |
all | 2 | 44 | 42 | 48 |
all | 3 | 46 | 49 | 50 |
all | 4 | 47 | 55 | 51 |
all | 5 | 48 | 60 | 52 |
all | 6 | 49 | 64 | 54 |
all | 7 | 50 | 68 | 55 |
all | 8 | 52 | 72 | 56 |
all | 9 | 53 | 75 | 57 |
Then we need raw-scores from a scale. If they do not exist, you may
use the score_scales
function to add sum scores. Therefore
set the sum argument to TRUE
. By setting
max_na = 0
, we do not allow missing values in any scale
item:
dat$raw_int <- score_scale(dat, subscale == "Int", sum = TRUE, max_na = 0)
dat$raw_ext <- score_scale(dat, subscale == "Ext", sum = TRUE, max_na = 0)
By default, lookup_norms
looks for T values:
dat$T_int <- lookup_norms(dat$raw_int, normtable = ex_normtable_int)
dat$T_ext <- lookup_norms(dat$raw_ext, normtable = ex_normtable_ext)
But this can easily be changed to percentile ranks, if included:
dat$PR_int <- lookup_norms(dat$raw_int, normtable = ex_normtable_int, to = "PR")
dat$PR_ext <- lookup_norms(dat$raw_ext, normtable = ex_normtable_ext, to = "PR")
T_int | T_ext | PR_int | PR_ext |
---|---|---|---|
43 | 40 | 35 | 14 |
43 | 46 | 35 | 49 |
47 | 47 | 55 | 53 |
71 | 87 | 95 | 100 |
47 | 64 | 55 | 89 |
44 | 50 | 42 | 64 |
61 | 40 | 88 | 14 |
46 | 46 | 49 | 49 |
53 | 62 | 75 | 87 |
47 | 77 | 55 | 98 |